Overview
Our research addresses physical climate processes with a focus on clouds, aerosols, their interactions (aerosol-cloud interactions) and response to global warming (cloud feedbacks).
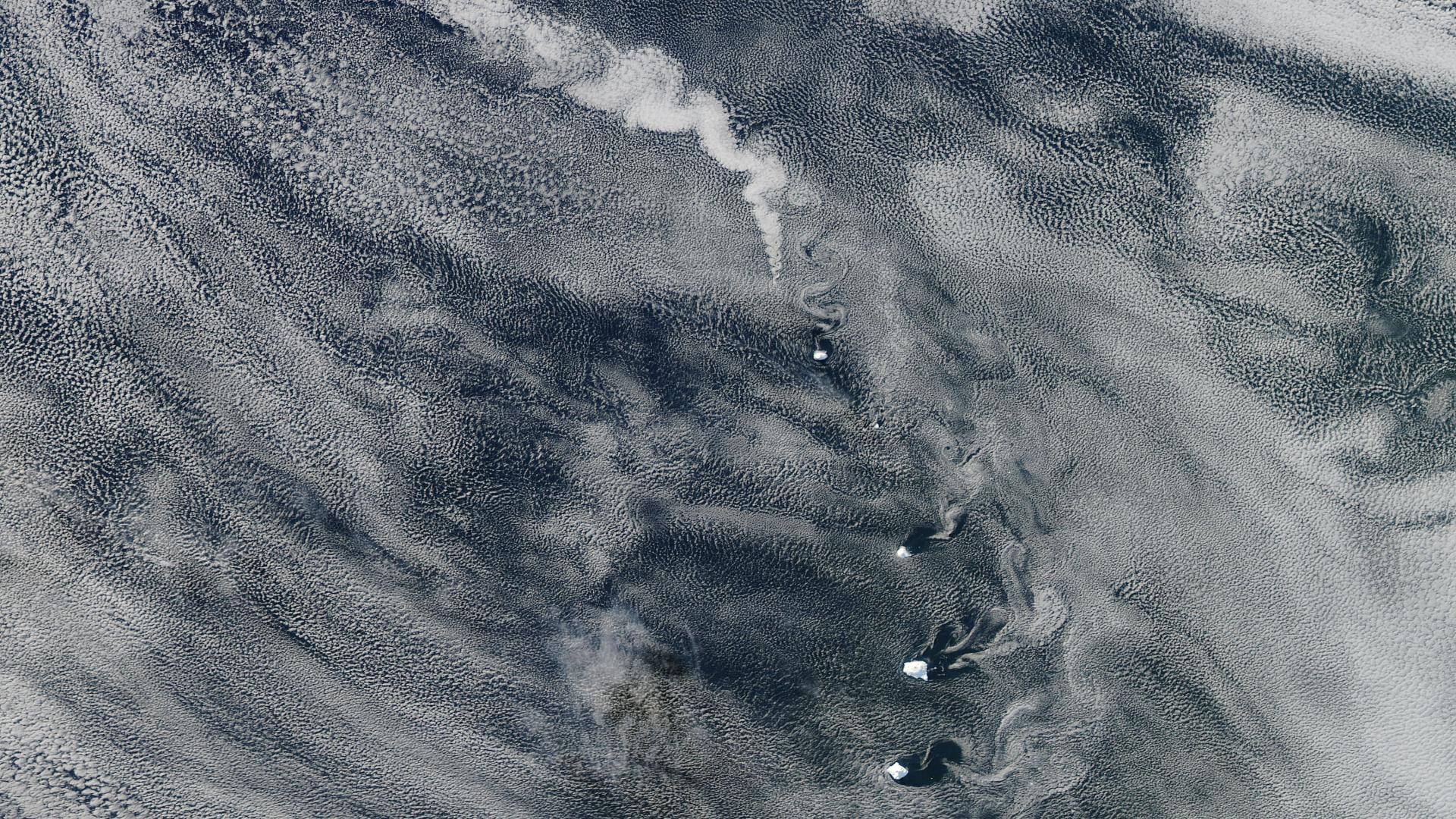
von Kármán vortex streets formed in the wake of volcanoes South Sandwich Islands. The brightening in the wake of a volcano emitting sulphate aerosol (precursors) demonstrates the cloud brightening albedo effect of aerosols, cooling the climate system.
Methods
Owing to the complexity of clouds and aerosols in the climate system, spanning scales from nanometers to thousands of kilometres, none of the sub-disciplines of Theory, Modelling and Observations have led to a significant reduction of uncertainties in our understanding of their role in the climate system, despite significant research over decades. In the Climate Processes group we strive to bridge these gaps and combine modelling closely with observational constraints – increasingly using machine learning.
Cloud resolving modelling
We employ high resolution cloud resolving models (CRM) and large eddy simulations (LES) for detailed process studies on clouds and their response to aerosols and global warming.
Movie: Simulation of convection over the Amazon using the ICON cloud resolving model (Herbert et al., JGR, submitted). Surface windspeed (blue-green) with overlaid precipitation (yellow-red) illustrate the important role of cold pools from (negatively buoyant) downdrafts in precipitating areas spreading across the surface. (Ross Herbert).
Climate modelling
We develop, evaluate, constrain and use climate models (ECHAM-HAM, ICON-HAM, HadGEM-UKCA, UKESM) to understand the climate response to aerosols and global warming with a particular focus on the hydrological cycle.
Observations
We use vast amounts of in-situ and satellite observations for process studies as well as to constrain clouds and aerosols in our modelling systems.
Machine learning
We increasingly use machine learning methods to analyse Earth Observations and to improve atmospheric models and our understanding of their uncertainty.
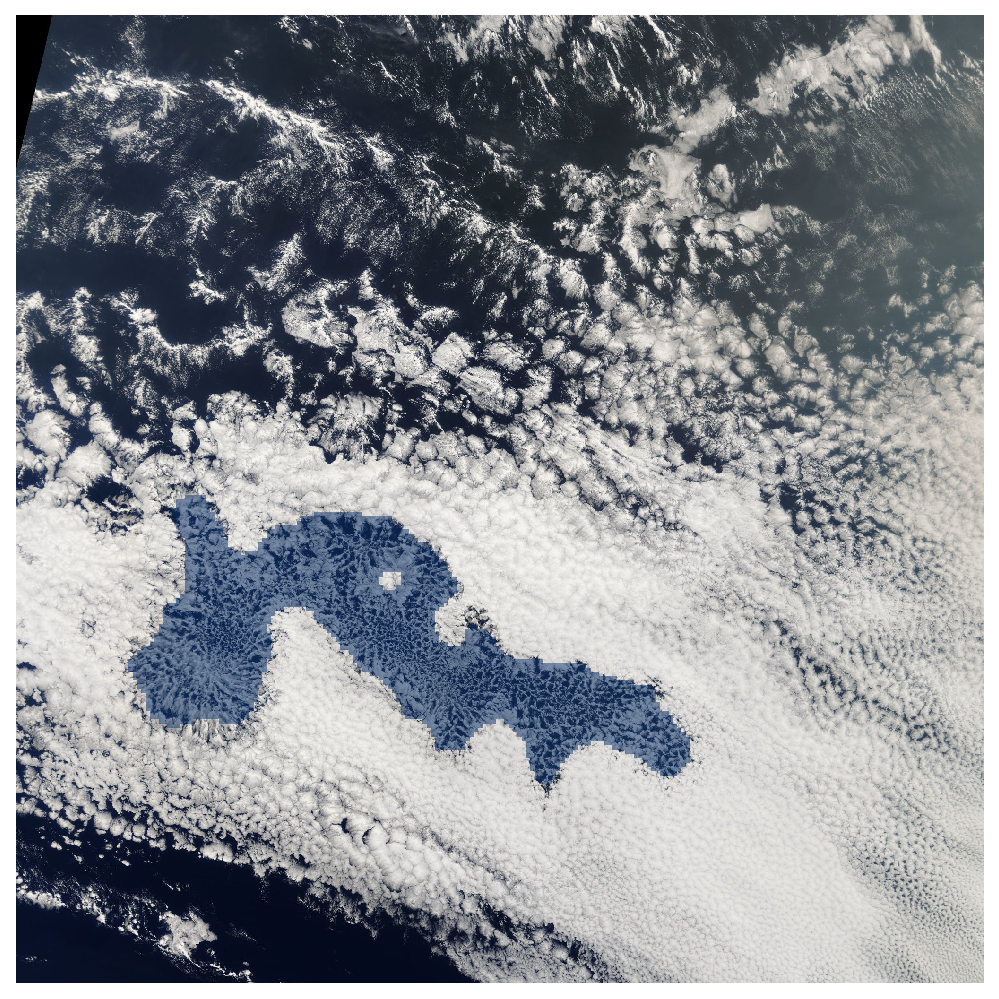