Seamless Predictions
Seamless predictions consider weather and climate forecasts from a range of prediction time scales using the same class of forecast models. Several of our below listed Research Themes make use of these connections across timescales:
- Extreme events and the role of climate change
- Multidecadal variability in seasonal forecasting skill
- Improving forecast using Machine Learning
- From forecasts to applications: seamless climate predictions
- Climate computing
- Model uncertainty
Extreme events and the role of climate change
Extreme weather and climate events, such as heat waves and windstorms, are very impactful meteorological hazards affecting people and societies. Our research focussed on the atmospheric and climate physics underlying these extreme, with a particular emphasis on the role of anthropogenic climate change.
These extreme events are amongst the most challenging aspects of the weather and climate system to accurately represent in numerical models, as they often involve small-scale processes that are poorly resolved in models. To address these challenges, we use state-of-the-art operational weather and climate forecast models that are demonstrably able to simulate the extremes in question.
Examples of cases that we have studied can be found in the following publications:
- Leach, N., A. Weisheimer, M.R. Allen, and T.N. Palmer (2021). Forecast-based attribution of a winter heatwave within the limit of predictability. PNAS, https://doi.org/10.1073/pnas.2112087118
- Ermis, S., Leach, N.J., F. Lott, S. Sparrow and A. Weisheimer (2024). Event attribution of a midlatitude windstorm using ensemble weather forecasts. Env. Res.: Climate, 3,035001,doi:10.1088/2752-5295/ad4200
- Leach, N.J., C.D. Roberts,M. Aengenheyster, D. Heathcote, D.M. Mitchell, V. Thompson, T.N. Palmer, A. Weisheimer and M.R. Allen (2024). Heatwave attribution based on reliable operational weather forecasts. Nature Commun., 15,4530,doi:10.1038/s41467-024-48280-7
People: Nick Leach, Shirin Ermis, Antje Weisheimer, Tim Palmer
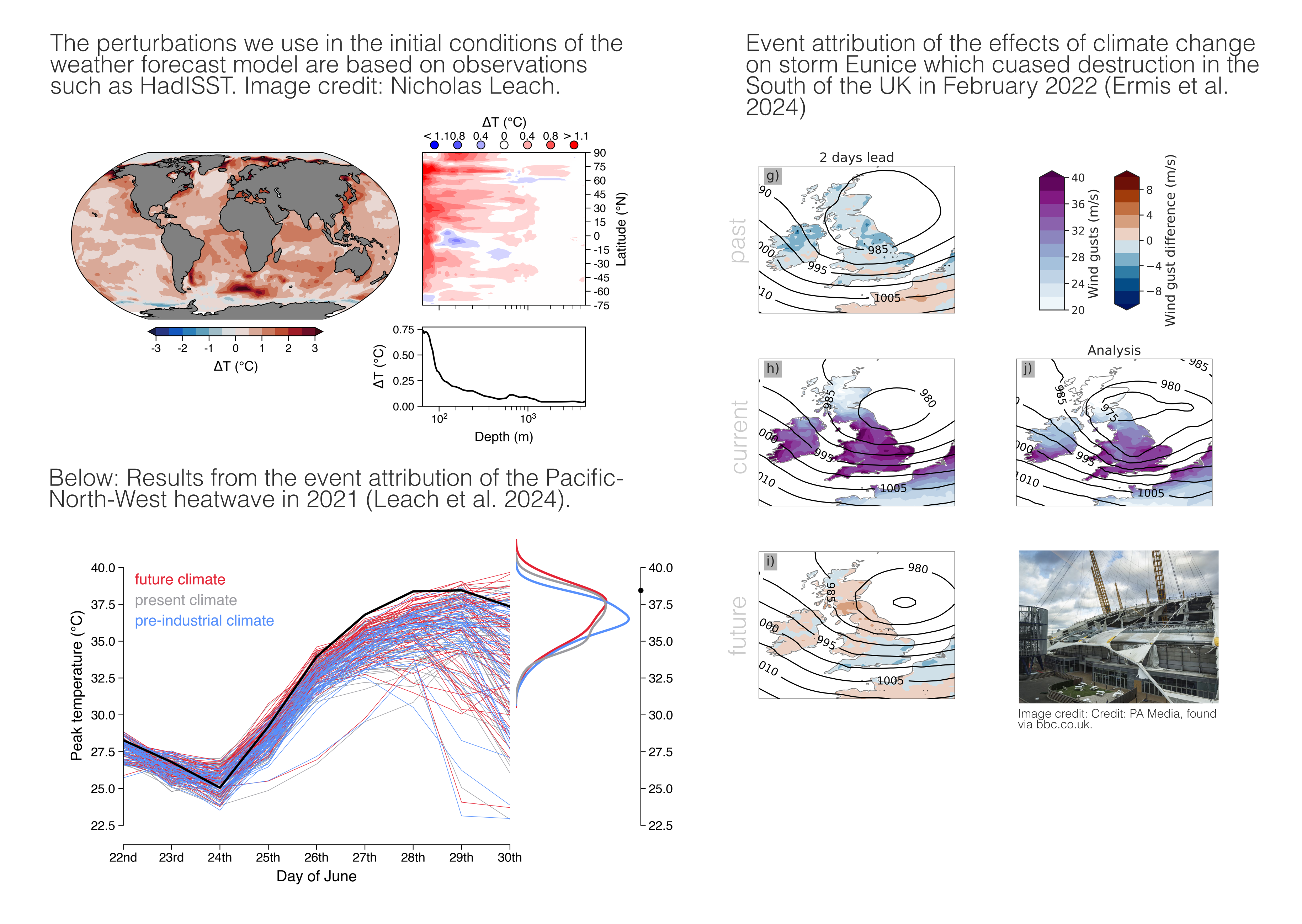
Multi-decadal Variability in Seasonal Forecasting Skill
Seasonal forecasting provides critical information for sectors including energy, agriculture, and natural disaster early warning systems. However, the skill of seasonal forecasts varies over time, influenced by both natural climate variability and external forcings. Our research in this area focuses on understanding multi-decadal variations in the seasonal forecasting skill of large-scale climate indices, including ENSO, the PNA, and the NAO. We are particularly investigating how changes in oceanic and atmospheric conditions affect predictability on seasonal timescales.
Furthermore, we study the role of atmospheric aerosols in seasonal forecasting models, quantifying their impact on key climate variables and forecast performance. Aerosols influence the radiative balance and circulation patterns, yet their representation in seasonal prediction models remains a source of uncertainty. By examining different aerosol forcing scenarios in seasonal hindcasts, we aim to improve our understanding of their contribution to seasonal predictability.
Publications:
- Wright, M.J., A. Weisheimer and T. Woollings (2024): Multi-decadal skill variability in predicting the spatial patterns of ENSO events. Geophys. Res. Lett., https://agupubs.onlinelibrary.wiley.com/doi/10.1029/2023GL107971
- Wright, M.J (2024): An introduction to weather, climate and the energy sector. Weather, https://rmets.onlinelibrary.wiley.com/doi/10.1002/wea.4612
- Weisheimer, A., N. Schaller, C. O'Reilly, D. MacLeod and T.N. Palmer (2017). Atmospheric seasonal forecasts of the 20th Century: multi-decadal variability in predictive skill of the winter North Atlantic Oscillation and their potential value for extreme event attribution. Q. J. R. Meteorol. Soc., doi:10.1002/qj.2976
- Weisheimer, A., D. Decremer, D. MacLeod, C. O'Reilly, T. Stockdale, S. Johnson and T.N. Palmer (2018). How confident are predictability estimates of the winter North Atlantic Oscillation? Q. J. R. Meteorol. Soc., doi:10.1002/qj.3446
- Weisheimer, A., D. Befort, D. MacLeod, T.N. Palmer, C. O'Reilly and K. Strommen (2020). Seasonal forecasts of the 20th Century Bull. Amer. Meteor. Soc., doi:10.1175/BAMS-D-19-0019.1
People: Matthew Wright, Antje Weisheimer, Tim Palmer
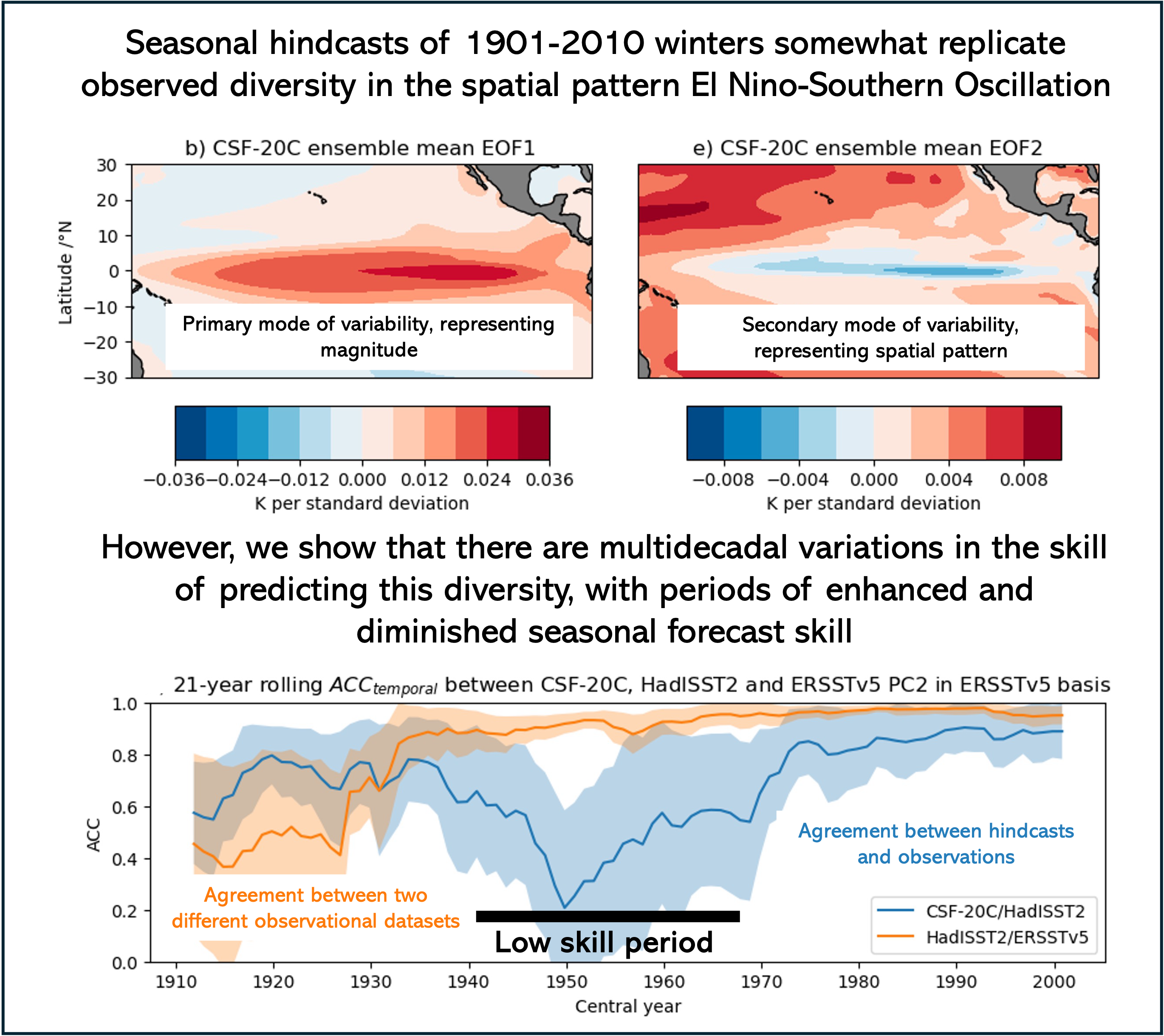
Improving forecasts using Machine Learning
Weather models derived from known laws of physics are run on enormous supercomputers at forecast centres such as ECMWF. Despite a quiet revolution in their accuracy, there are still systematic errors in their output. These errors are due to a lack of computing power, imperfect representation of physical processes or systematic errors in the measurements used to constrain them. By comparing these errors to what observations say really happened, we can find out when they occur and introduce a correction to future forecasts. We develop statistical machine-learning methods to automate this process.
Papers:
- Harris, L., McRae, A. T. T., Chantry, M., Dueben, P. D., & Palmer, T. N. (2022). A generative deep learning approach to stochastic downscaling of precipitation forecasts. Journal of Advances in Modeling Earth Systems, https://doi.org/10.1029/2022MS003120
People: Fenwick Cooper, Shruti Nath, Charlotte Merchant, Antje Weisheimer, Tim Palmer
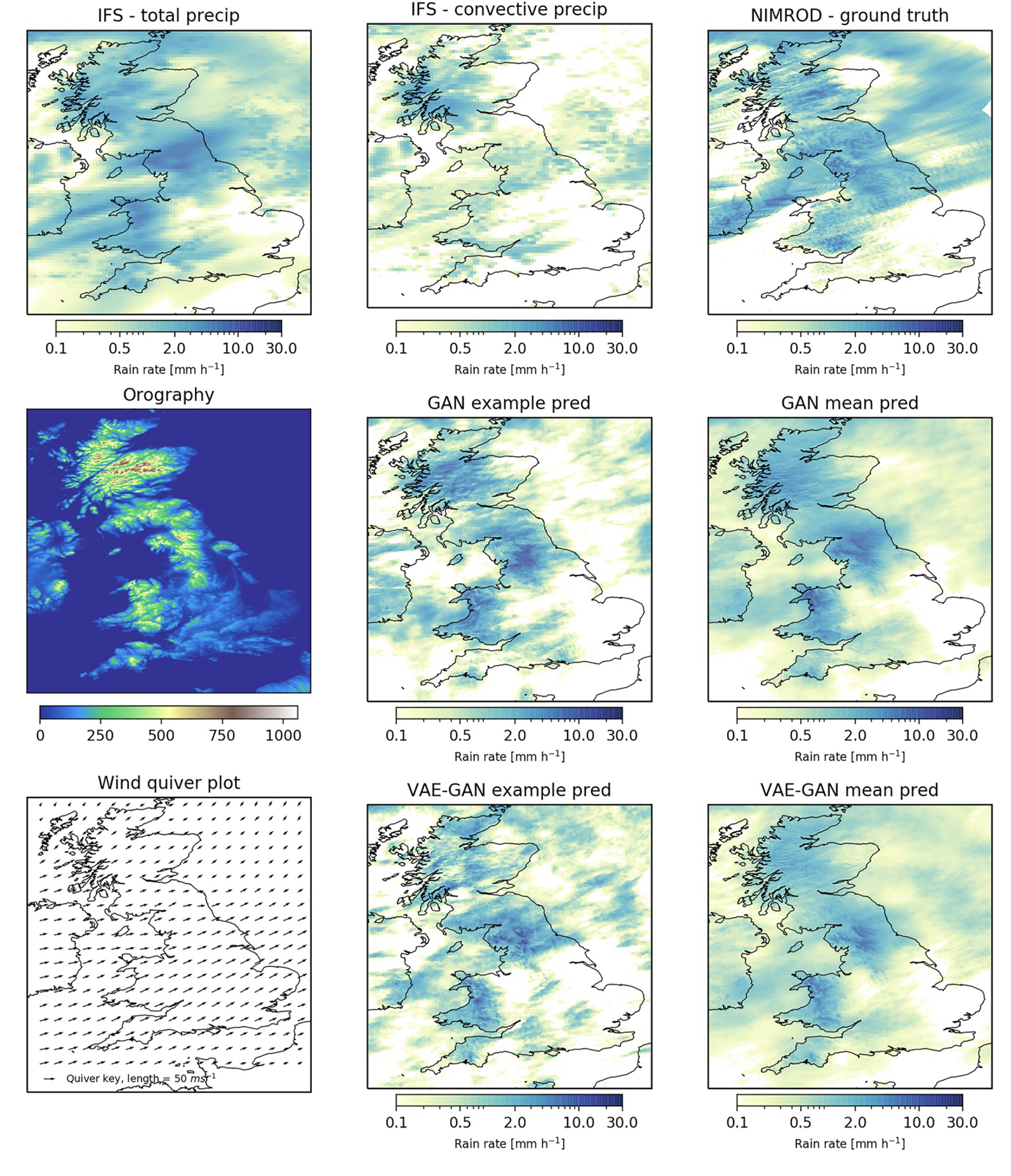
Generative adversarial network (GAN) and variational auto-encoder-generative adversarial network (VAE-GAN) model output example predictions and means from 09:00-10:00 UTC of the 9 February 2020. Shown is the total precipitation, wind direction and strength from the IFS forecast from ECMWF, orography, and the ground truth: NIMROD radar data.
From forecasts to applications: seamless climate predictions
Numerical weather and climate predictions exist across various time scales, ranging from days and weeks to seasons, years, and decades. While these forecasts are based on similar global circulation models of the coupled atmosphere-ocean-sea-ice-land system, they are often not "seamless", meaning the forecasts do not consistently cover all time ranges. Instead, primarily for practical reasons, forecasts are typically produced separately for different time scales, such as medium-range, sub-seasonal, seasonal, multi-annual, and decadal forecasts. As a result, forecast users find it challenging to use these different products when their needs span a range of forecast time scales.
As part of the EU-funded project ASPECT, we are researching ways to effectively combine and merge different forecast products into a seamless prediction framework. This approach is designed for users who require continuous climate prediction information for seamless decision-making. A unique element of the ASPECT project is the co-production of user needs by understanding user requirements and developing tailored methods that support value-added, user-relevant adaptation strategies.
Key users of ASPECT include organisations from agriculture, governance, finance, humanitarian, and disaster-response sectors, all of which have been significantly impacted by climate extremes in recent years. We are developing novel temporal merging methods to provide seamless climate information across the monthly to multi-annual time scales for tailored climate extremes. The current focus is on spring frost risk for Spanish vineyard farmers in Catalonia, and extreme hot weather risk for the British Red Cross in the UK.
Publications (from a previous project):
- Befort, D.J., L. Brunner, L.F. Borchert, C.H. O'Reilly and A. Weisheimer. (2022). Temporal combination of decadal predictions and climate projections: challenges and potential solutions. Geophys. Res. Lett., doi:10.1029/GL2022098568
- Befort, D.J., C.H. O'Reilly and A. Weisheimer (2020). Constraining projections using decadal predictions. Geophys. Res. Lett., doi:10.1029/2020GL087900
People: Muhammad Adnan Abid, Beena Balan Sarojini, Antje Weisheimer
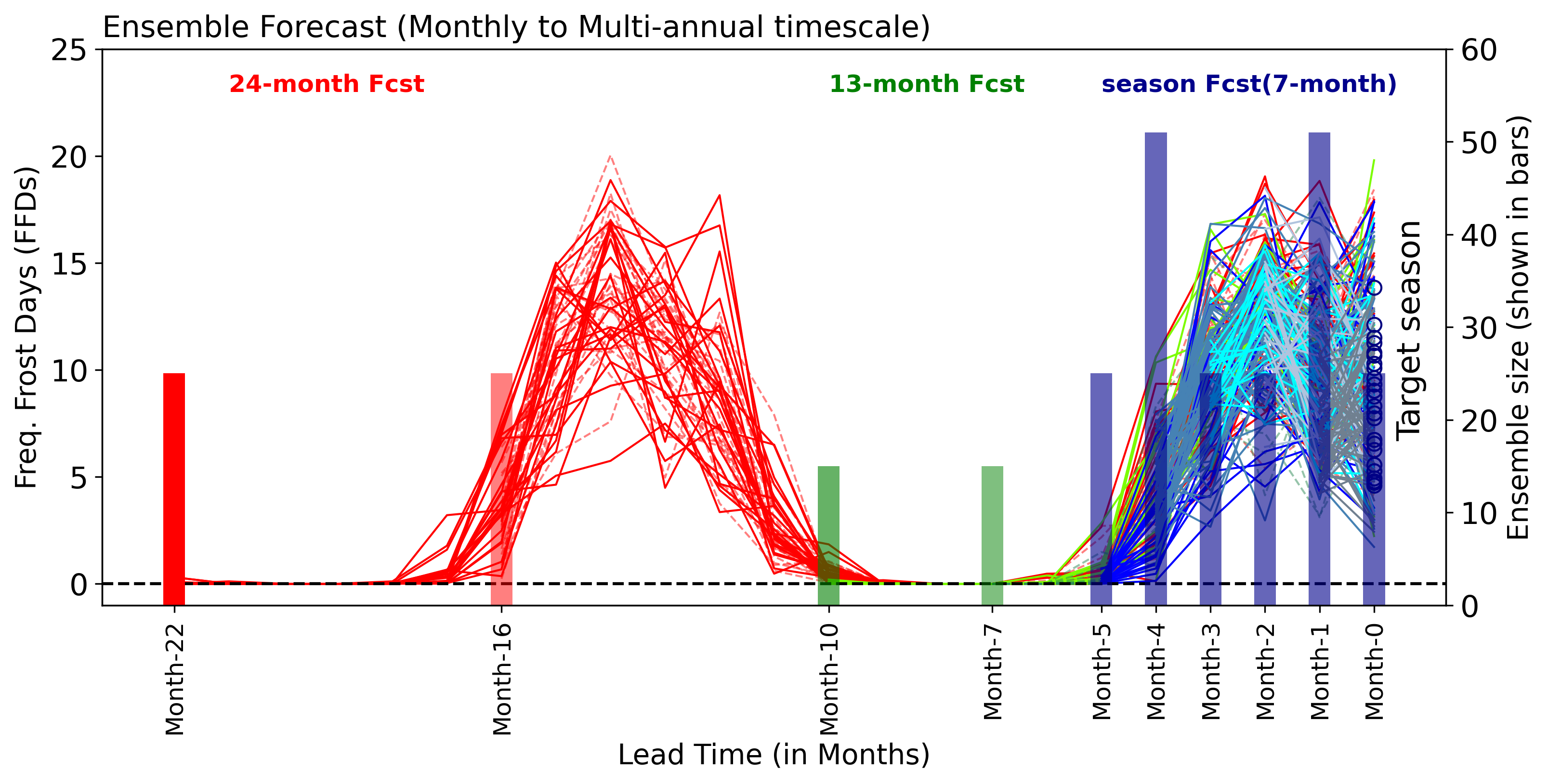
The figure illustrates the temporal merging concept for frost day forecasts over the Catalonia region. Forecasts shown as trajectories are from 7-month (blue), 13-month (green) and 24-month (red) time scales using the ECMWF seasonal forecasting system SEAS5. Bars represent ensemble size for forecasts available from individual lead time within those time scales.
Climate computing
The intricacy of the earth system, coupled with the multi-scale nature of climate, makes its prediction one of the most computationally complex problems in science. The fidelity of climate simulations depends on both physical model accuracy and computational efficiency. However, insufficient model resolution, constrained by computational cost, limits how effectively model uncertainties can be mitigated. Advances in computing enable performance gains to be reinvested in higher resolutions, larger ensembles, and improved uncertainty representation, ultimately enhancing predictive skill.
Historically, efforts have focused on improving computational efficiency through reduced-precision numerics and investigating the potential of quantum computing. Currently, we are exploring machine learning techniques to accelerate high-resolution climate modeling and maximize the information extracted from physics-based simulations.
Selected publications:
- Stevens, B., Adami, S., Ali, T., et al. (2024). Earth Virtualization Engines (EVE). Earth System Science Data, 16, 2113–2122. https://doi.org/10.5194/essd-16-2113-2024.
- Tennie, F., and T. N. Palmer (2023). Quantum Computers for Weather and Climate Prediction: The Good, the Bad, and the Noisy. Bull. Amer. Meteor. Soc., 104, E488–E500, https://doi.org/10.1175/BAMS-D-22-0031.1.
- Paxton, E. A., M. Chantry, M. Klöwer, L. Saffin, and T. Palmer (2022). Climate Modeling in Low Precision: Effects of Both Deterministic and Stochastic Rounding. J. Climate, 35, 1215–1229, https://doi.org/10.1175/JCLI-D-21-0343.1.
People: Charlotte Merchant, Felix Tennie, Antje Weisheimer, Tim Palmer
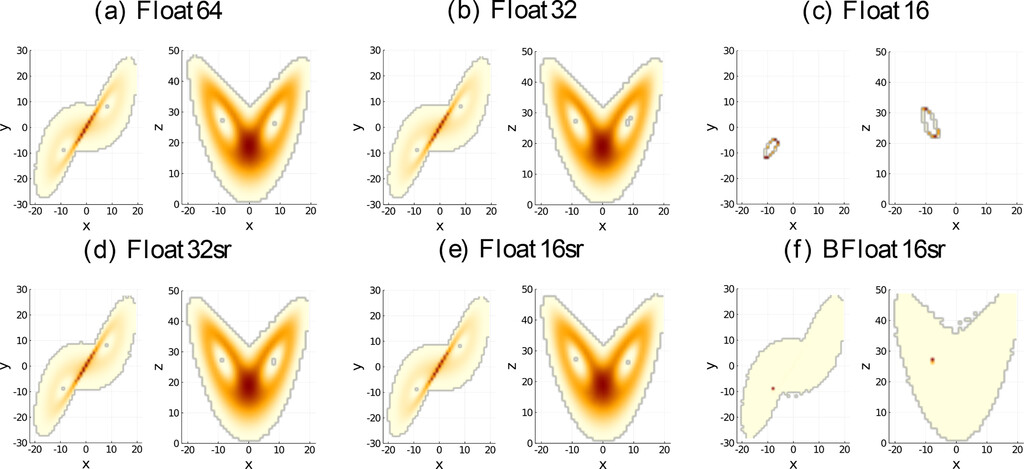
From Paxton et al. (2022)
Model Uncertainty
Optimal representing model uncertainty is a key challenge in climate modelling. Explicit representation of sub-gridscale uncertainties has been shown to provide a wealth of benefits to forecasts; reducing model biases, improving skill and fundamentally giving better estimates of forecast uncertainty along with the forecast. We study the impact of different methods of model uncertainty representation, particularly stochastic physics, and are in the process of developing new methods.